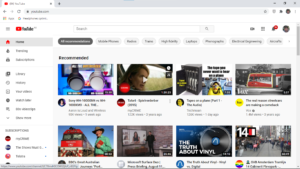
Content recommendation engines on the likes of YouTube can easily lead viewers down a content rabbit hole if they are not careful
Article
This website lets you see how conspiracy theorists fall down the YouTube rabbit hole | Mashable
My Comments
Increasingly a lot of online services, be they social media services, news-aggregation portals, video streaming services and the like, are using algorithms to facilitate the exposure of undiscovered content to their users. It is part of their vision to effectively create a customised user experience for each person who uses these services and is part of an Internet-driven concept of “mass customisation”.
Those of you who use Netflix may find that your newsletter that they send you has movie recommendations that are based on what you are watching. You will also see on the Netflix screen a “recommendations” playlist with movies that are similar to what you have been watching through that service.
A very common example of this is YouTube with its recommended-content lists such as what to view next or what channels to subscribe to. Here a lot of the content that is viewed on YouTube is the result of viewers using the service’s personalised content recommendations.
The issue being raised regarding these algorithms is how they can perpetuate a personal “thought bubble”. It is even though there is other material available on the online service that may not mesh with that “bubble”. Typically this is through surfacing content that amplifies what the viewer has seen previously and can pander to their own biases.
An online experiment created by a Web developer and funded by the Mozilla Foundation explores this concept further in context with YouTube. This experiment, called “TheirTube”, emulates the YouTube content-discovery and viewing habits of six different personalities like conspiracists, conservative thinkers and climate deniers when they view content related to their chosen subjects.
Here, it shows up what is recommended in relationship to content to view next or channels to subscribe to for these different personalities and shows how the content recommendation engine can be used to underscore or amplify particular viewpoints.
It is a common problem associated with the artificial-intelligence / machine-learning approach associated with content recommendation that these services use. This is due to the end-user “seeding” the algorithms with the content that they actually interact with or the logical content sources they actually follow. Here, the attributes associated with the content effectively determine the “rules” the algorithm works on.
If you are trying to maintain some sort of critical thinking and use content services like YouTube for informational content, you may have to rely less on the content-recommendation engine that they use for finding new content. You may find it useful to manually seek out content with a contrasting viewpoint to avoid the creation of a “thought bubble”.
As well, if you follow the online-service’s recommendations in addition to running contrasting content through the online service, you may be in a position to make the content recommendation engine bring up varied content.
The idea of content recommendation engines that are based on what you choose can allow us to be easily cocooned in a content bubble that perpetuates personal biases.