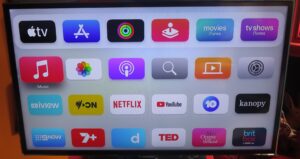
Will free-to-air TV apps be required to have pride of place on your connected TV device’s home screen?
There is a fight brewing between the established free-to-air TV lobby and the pay-TV / subscription video-on-demand lobby about what should take the primary position on connected-TV devices. It is part of a continual debate regarding the continued existence of the free-to-air TV services with other issues surfacing like anti-siphoning protection so that the sports that matter don’t end up exclusively on pay-TV or online subscription services.
This is more intense in countries like the United Kingdom, Europe and Australia whose governments and society place strong importance on the established public-service, commercial and community free-to-air TV services and their continual role in informing and entertaining us.
It is although younger generations are drifting away from these services towards the likes of Netflix or YouTube for their entertainment. This has been driven by the “cord-cutting” movement in the USA where younger people are ditching their cable or satellite pay-TV subscriptions in favour of online video services.
Why protect established free-to-air TV?
There is a strong societal defence for traditional established free-to-air TV in the UK, Ireland, Europe, Australia and similar countries. This is because the national free-to-air TV platform is based around at least one popularly-accepted and well-respected public-service broadcaster and a handful of commercial free-to-air broadcasters. In a lot of cases, most of the TV services existed since TV was commercialised in the Western world with some services, especially the public-service broadcasters, existing at the dawn of regular radio broadcasting in that area.
As well, due to the fact that the free-to-air TV broadcasters have to have a government-issued license or public-service-broadcasting charter to operate on the VHF and/or UHF wavebands, there has been strong human oversight over the content they publish and that it matches current social standards. This has led to it being considered a socially respectable platform and broadcasters who run advertising know that they are operating a brand-safe platform for their advertisers.
People who live in nations that value free-to-air TV see the free-to-air broadcasters as offering more locally-produced content due to various local-content mandates associated with their licences or charters. They notice that the free-to-air broadcasters play a significant part in boosting their nation’s cultural “soft power” and identity on the world stage. For example, there are shows like ABC Australia’s “Bluey” or Network 10’s “Neighbours” or “MasterChef Australia” that have acquired significant international viewership because they represent that Australian camaraderie.
Free-to-air TV broadcasters, especially the public service broadcasters like the BBC or Australia’s ABC are being valued as reliable sources of news and information. This is more so in the era of “post-truth” where fake news and disinformation delivered by social media is muddying the waters about what is accurate information or not and established media is seen as the preferred go-to if you are after accuracy in your news.
The debate is also about an existential threat to established media including free-to-air TV from Silicon Valley. This is because the online services offered by Silicon Valley, including social media, are being seen as offering newer fresher content than what established media can offer, something valued by younger people.
The current situation with connected TV devices
Connected TV platforms, whether represented by a Smart TV, a set-top box or a streaming stick, place importance on the home screen that these devices show up when you turn them on initially or press a “home” button on their remote control. These platforms are driven by client apps for various video-on-demand or streaming-TV services with users having to use the platform’s app store to install these client apps for the services they use.
Such services are represented by the likes of Netflix, YouTube or Vimeo; but also include local subscription-funded or advertising-funded video-on-demand services. This may include the classic local pay-TV platforms that have set themselves up to deliver via Internet in addition to or in lieu of their legacy satellite or cable means, using an app rather than a set-top box for access to their content. Then most of the connected TV platforms have links to their own transactional or advertising-driven video-on-demand facility, FAST (free ad-supported streaming TV) service, video game store or other services.
In addition, some connected TV devices have dedicated buttons on their remote controls to facilitate one-touch access to video services like Netflix or YouTube. This is part of a partnership between the connected TV platform and the video service provider to maintain that kind of access.
To preserve their relevance in the connected-TV era, established free-to-air TV broadcasters are offering Broadcaster Video-On-Demand apps which make their content available on-demand This is based on previous “catch-up TV” services where you can catch up on prior episodes of a TV series, but now offer extras like binge-view opportunities or supplementary content.
Some broadcasters even offer free streaming TV channels through these apps that they wouldn’t be able to offer via RF means. This would include access to versions of their channels that are editorially different in other parts of the nation, such as to allow travellers and the like to follow content in their home area. Or they would include special-event channels like showing each sport in the Olympic Games as separate channels. They may even stream channels from other content providers they have a strong business relationship with like what the Paramount-owned free-to-air channels are doing by hosting at least some of the Pluto TV FAST channels on the 10Play and My5 BVOD apps.
Even some of the advertising on content viewed through these apps has interactive TV elements such as “shoppable” advertising or access to long-form video content. This is in addition to experiencing a reduced ad load during the commercial breaks with the ads being more relevant to you, when you watch a show on demand through these services. But the important fact with the advertising is that the broadcasters control and benefit from the commercials that appear.
But there are a significant number of connected TV platforms that require the user to download the BVOD apps from the platform’s app store before you can use them. Then these apps typically end up at the bottom of the home screen and you would have to move them around to be visible when you turn the TV device on.
This may be an exception with some Smart TVs and RF-tuner-equipped set-top devices sold in the UK, Australia and New Zealand that implement the HBBTV-enhanced Freeview electronic programme guide that supports the ability to see prior shows. These have free-to-air BVOD apps pre-installed so as to facilitate this kind of viewing from the EPG.
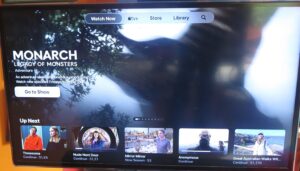
Will connected TV platforms be required to recommend content from established free-to-air channels here?
An increasing number of connected TV platforms are integrating “one-stop” content search, “next-episode” and content recommendation functions that tie in with their various video-on-demand apps and platforms. This allows a viewer to search for a particular show or identify shows worth watching at one point rather than diving in to and out of the various apps to find a desired programme. This is facilitated through the use of application-programming interfaces that provide a link to the content services via their apps.
Other upcoming trends to impact connected TV
The UK and Australia at least see the distribution via RF means using the outdoor aerial as the universal standard for access to free-to-air TV. This is although Continental Europe and, to some extent, Canada place acceptance on other RF-delivery means like cable or satellite delivery as part of universal access to free-to-air TV.
Free-to-air TV via the Internet or your home network
But the DVB Forum have established standards for delivery of TV service including free-to-air TV via local-area-network and Internet setups. One of these is DVB-I which is about streaming from Internet servers hosted by the broadcasters themselves while the other, known as DVB-HB, is about streaming from a broadcast-LAN tuner device connected to an aerial, cable-TV setup or satellite dish via a local area network. Both these systems provide the same user experience as traditional RF-based TV setups such as the ability to “channel surf” with the remote control.
This is in addition to the broadcast video-on-demand apps offering access to the broadcaster’s own free-to-air offerings and extra free streaming TV offerings through the Internet. In many cases, this also includes an electronic programme guide for these online channels, You may even find that you have access to area-specific content like news bulletins from other locations. But these apps don’t necessarily offer the classic user experience associated with watching TV.
Internet-first TV devices and setups
Your laptop, tablet or smartphone are replacing the traditional small portable TV as the way to watch TV in areas like the kitchen or garage. This is thanks to the broadcast video-on-demand platforms being available as an app or Website for regular or mobile computing platforms.
Add to this the smart display platforms like Amazon Echo Show or Google Nest Hub where you can summon particular channels using your voice. LG and Samsung haven’t forgotten the dot-com-era idea of Internet fridges with their products that could have support for video apps including free-to-air BVOD apps.
Subsequently LG and Samsung have been offering Internet-first TV devices, also known as smart monitors. LG has been offering the StanByMe range of monitor-sized battery-operated lifestyle TVs with one that folds up in to a suitcase. Samsung has also offered their Smart Monitors which combine the smart-TV functionality in to a computer monitor but don’t provide the RF tuner. It also includes people using connected-TV devices without RF connection to an aerial to watch their TV content, thanks to BVOD or TV-over-IP apps.
Here, these are devices that implement computing, smart-display or Smart TV platforms including access to video-on-demand platforms but omit RF tuners. With them, you could pull in video content via apps whether it be streaming in real time or playing from a video-on-demand server. In the case of the Internet-first TV devices, they would have a remote control so you can select what you want to view or regulate the sound from afar.
Delivery to airliners and ships
In some countries like India, it has been proven that they could use higher-bandwidth satellite Internet to deliver live free-to-air TV from the local TV channels to airliners flying domestic flights and offer this as a form of live inflight entertainment. Such setups would come in to their own with the sports that matter where people want to follow those matches during their flight.
This is facilitated with current-generation high-bandwidth satellite broadband Internet that is being delivered to commercial jets equipped with the necessary technology. More of these jets are being equipped for Wi-Fi Internet from the satellite broadband typically to provide as a passenger amenity whether bundled as part of a premium airfare or purchased at an extra-cost option. In a similar context, this could appeal to the maritime cohort where the passengers onboard ferries, cruise ships, yachts and the like, or the crew within navy or merchant-navy ships could gain access to free-to-air TV via Internet-based delivery thanks to satellite or other means.
Here, the combination of Internet-based TV service delivery and these satellite Internet services could make it feasible to have live free-to-air TV from local TV stations delivered as an entertainment option for air or maritime travel. Add to this the ability to use BVOD apps to allow air or ship passengers to watch shows from these stations on demand.
The issues here
The free-to-air TV lobby had noticed that connected TV platforms are engaging in some form of “pay to play” when it comes to what appears on the home screen by default. Here, it was perceived as being financially and ethically difficult for local free-to-air broadcasters to get their BVOD apps on the home screen within their country of operation.
Here. the local free-to-air TV establishment want the national governments to use legislation or regulation to make sure that their BVOD apps are installed by default and easily discoverable. This includes a similar want for content offered by free-to-air broadcasters, preferably locally-produced content, to be brought forward in any platform-wide content-search or content-recommendation engine.
On the other hand, the pay-TV lobby who are also representing subscription video-on-demand or even Big Tech want to maintain the status quo “in the name of the consumer”. This is due to a perceived fear that the customer who currently subscribes to an online video service not offered by free-to-air TV broadcasters won’t be able to install or satisfactorily use any client-side apps for these services. This includes not being able to see the online video service’s content in search results or content recommendations.
I see this argument of relevance when it comes to content search and recommendation engines that are being built in to the connected TV platforms. As well, this may impact one-touch access buttons on remote controls where it wouldn’t be economically feasible to provide this kind of access to any online video service, whether free-to-air or paid.
Which kind of devices will this affect?
Primarily I see this requirement affect TVs and set-top devices including streaming sticks pitched for household use. This is because these devices are primarily marketed for watching video entertainment in the home.
But there will be a call for Internet-first TV devices like the LG StanByMe family or the Samsung Smart Monitor family to face this requirement because of them being marketed for video entertainment consumption as a key use case.
Computers, mobile platform devices and games consoles may not face this requirement due to their core use case not being video entertainment. This is although you can use them to enjoy video entertainment using Websites or apps. But you may find that companies selling tablets or 2-in-1 laptops will want to court the countries that value free-to-air TV as an entertainment source by packaging the likes of BBC iPlayer, ITVX, ABC iView and 10Play alongside Netflix or YouTube when users register these devices in those countries. Or the app stores simplify the process of installing these BVOD aps.
A question that can come up is whether this requirement will only be for TVs and set-top devices sold primarily for household use. This is because TV manufacturers also sell a separate range of “commercial use” TVs that are pitched for installation in hotels, bars, common rooms, hospitals and the like. Here, we are likely to use these sets to watch TV content away from home such as in our hotel rooms and we would like the same user experience that we have at home.
These sets and devices are likely to have extra programmability in order to satisfy particular use cases like hotels. The software may be updated at a later time or manually compared to what is installed on equipment for the home and there may be a “business use” app store for apps used in a business sense. But there is a risk of manufacturers offering a Smart TV or set-top device as “commercial use” but sell them to householders to evade the various mandates associated with equipment sold to the residential market.
Small lodging places like bed-and-breakfasts / guest-houses, motels and inns are likely to prefer to purchase and install residential-use TVs. Similarly, houses or apartments available for short-term rental will prefer residential sets. This is due to the sets being cost-effective and offering a familiar user experience; and the businesses not wanting or needing superfluous levels of sophistication for their technology.
But could they be required to adhere to the same rules as residential-use equipment such as prominence for free-to-air apps on the home screen?
How does this impact connected TV device design and user experience
The impact of these mandates will affect the connected TV experience in a few ways,
When you set up a new device
Firstly, when you set up a new Smart TV or other connected TV device, you will find that the mandated apps are downloaded and installed by your device when it is connected to your home network. These will then appear on the home screen as part of the default setup, This is because of the fact that you have to select the country you’re in at or close to the setup / install routine and this determines what apps are downloaded and installed in your connected TV device.
This situation will also occur if you have to subject your connected TV device to a factory-reset due to it being balky or something you should do when you take possession of a second-hand device. Some devices may rearrange the screen for the mandatory apps and download or update them during a major software upgrade.
The home screen
Under these mandates, the home screen on a connected TV device will be required to have the free-to-air BVOD apps in the home screen and accessible with a minimum of scrolling.
Some home screens may create a “local TV” strip with the free-to-air BVOD apps inhabiting that strip on the home screen towards the middle. It most likely would exist alongside an icon that allows you to view an electronic programme guide and change channels if it has RF-based or IP-based tuning means.This will most likely be above a larger strip with Netflix, YouTube and other popular subscription services inhabiting that strip.
This is whereas others like Apple TV will simply sequence the free-to-air BVOD apps ahead of Netflix, YouTube and co. These may even have to group them on the same row and have each icon highlighted in a distinct manner.
You will still be able to use the “screen edit” function that your connected TV platform offers to rearrange the app icons on the home screen. This may be to bring all the services you use frequently and regularly closer to each other on the home screen. This kind of option may be either invoked through selecting a “screen edit” function on the setup menu or holding down on one of the icons to invoke “screen edit”.
Content search and recommendation behaviour
A mandate for free-to-air-TV prominence that impacts content search and recommendation would require a content search engine that works across a connected TV platform to place free-to-air TV content at the top of the “found content” list.
The content recommendation or “up next” (list episodes in a series that aren’t viewed)engine in the connected TV platform would also be required to place content from free-to-air broadcasters at the head of the recommended-content list. There may even be a requirement to place locally-produced content first, to encourage us to prefer this content for our evening’s viewing.
This would put the pay TV lobby’s nose out of joint because it would be hard to discover the content that a pay-TV or other online service has to offer. That is even though you have the likes of Netflix who are having their local offices commission original local work around the world and satisfy local content requirements for their social licence to operate in other countries.
One-touch access to services on the remote control
Another issue is how the remote control is designed if the manufacturer is to have one-touch buttons for access to particular online video services. If local free-to-air apps are being mandated as far as the remote control is concerned, it may be about adding five or more extra buttons with logos representing the online platforms offered by local free-to-air channels. This would be in addition to buttons for Netflix and YouTube,
Then the manufacturers forego the economies of scale associated with designing and manufacturing the same remote control with the same functionality and labelling for all world markets. Such an approach would also not survive any rebranding efforts that an online video provider undertakes.
This problem may be solved through implementing touchscreen or e-ink technology in remote controls. It also exploits the trend to use Bluetooth two-way communication in consumer-electronics remote controls so you are not having to have clear line of sight between the handset and the device.
It would come about with an array of six or eight e-ink-labelled buttons or a touchscreen that uses OLED or similar technology. The button array or touchscreen would be populated with the logos of the popular or mandated online video services for the country the device is used in and you use that to select these video services. Such a design may exist as a way to globalise the remote control design and bring back economies of scale by supporting “mass customisation”.
But this approach may require designers to go back to the traditional remote control design associated with TV sets and pay-TV set-top boxes rather than a very small “stick-like” design. This approach would come across as an approach that some consumers may prefer because it is a size that is harder to lose and would be welcome with devices that support traditional TV user interfaces.
What can be done
Customisation options
There is the issue of customising the home screen layout after you have set up your connected TV device. This is something you will have to do in order to make it easy to discover the services that you make use of, no matter what kind they are.
Here, any requirement to place free-to-air BVOD apps at the top of the home screen shouldn’t preclude you from rearranging your service icons around the screen. This may be due to you preferring and regularly visiting a particular mix of services whether free-to-air, classic pay-TV or online-first.
That means a connected-TV platform would have to have the home-screen customisation process assessed as part of its useability testing, with this assessed under “lean-back” operation conditions. That is where you are at a distance from the screen, typically in an armchair or couch, and using the standard remote control associated with the connected TV device.
The process could be simplified by “quick arrange” shortcuts like “Local TV first”, “Popular Online first” or “Frequently Used first” which sorts the icons according to particular orders.
These user customisations would need to be saved, whether on the device or on the user account associated with the connected TV platform.
Device-level / account-level content ranking preferences
There could be support for device-level or account-level learning of regularly used online video services. This would show up search results or recommended content results based on the services you have enrolled with and are frequently using.
Viewers should have the ability to enable ranking various factors such as services, locality of the content, content quality . Again these settings could be saved either on the device or the user account.
This could also be about connected TV platforms supporting the importing of watchlists from other sources. It could then allow film / TV experts and personalities that you follow to hand-curate and publish watchlists of recommended content so you can work through what they have recommended.
Conclusion
This debate over mandating free-to-air TV apps on connected TV devices will affect how they and their operating systems are designed. This will be about what appears on the home screen by default or which providers’ output appear at the top of platform-wide content search or recommendation results.